The Power of Deep Learning Neural Networks
Artficial neural networks are currently driving some of the most ingenious inventions of the century. Their incredible ability to learn from data and the environment makes them the first choice for machine learning scientists. Compared with traditional stochastic and mathematical methods, Artificial Intelligence enhances the out-of-sample predictability, it relies less on unrealistic assumptions and it learns complex, non-linear, and non-continuous interaction effects. This results in an improved signal-to-noise ratio and a much better capacity to decipher the complex market dynamics.
AI Science - Q&A
According to a broad scientific consensus we witness one of the largest scientific revolutions in mankind’s history. Artificial Intelligence is about to improve every aspect of our daily lives thanks to the outstanding work of responsible engineers and scientists. This scientific revolution also gives rise to a paradigm shift in the asset management industry toward a more mature, industrialised, digitized, systematic, and scientific way of constructing investment portfolios using the support of AI. This cutting-edge technology cannot only be employed to enhance returns, but also be used to manage risks. With the power of AI, entirely new ways of creating scenarios, simulating correlation matrices and (stress) testing portfolios are now available to professional investors. Thus, future regulatory requirements could be met, and sound risk management practices could be implemented. Examples are packaged retail investment and insurance products performance scenarios and European Securities and Markets Authority stress testing. As a consequence, similar to the obligation of minimising the total expense ratio (TER), of including ESG criteria and diversity industry standards, incumbent regulators such as the SEC, BaFin or FINMA may soon require professional investors to prove that they have thoroughly assessed the implementation of scientifically superior methodologies to assess product risks. AI-powered investment strategies are therefore poised for becoming rather an integral part of an investors’ investment style than just a niche asset class.
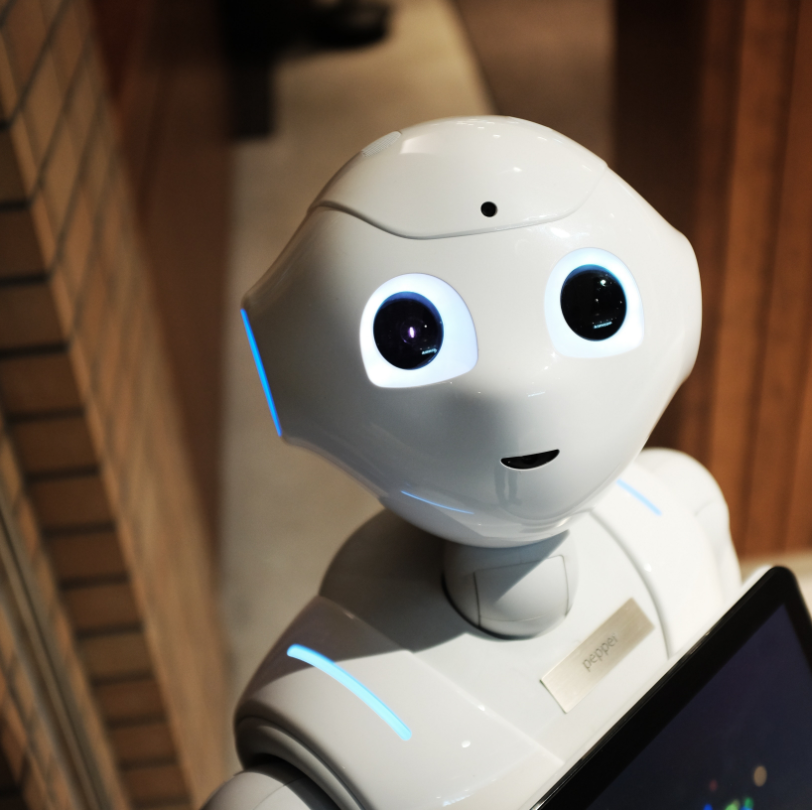
What is artificial intelligence (AI)?
Why AI has launched the next quant revolution?
Which types of AI data exist and what is its predictive power horizon?
Which AI-methods can be applied to analyze price data (financial time series)?
Can AI-powered algorithms predict financial time series?
Can AI improve the optimal portfolio construction?
Does AI enhance the tools for financial risk management?
Can AI generate better option pricing estimates than Black-Scholes?
What is Natural Language Processing (NLP)?
Which Deep Learning Models suit best for NLP?
Why NLP matters in asset management?
What are Convolutional Neural Networks (CNN)?
Why CNN can be employed to visualise fundamental or unstructured data?
How can Audio Signal processing filter the core information of financial time series?
How can Knowledge Graphs bring context to AI?
Will AI change the asset management industry?
Will humans become obsolete in the asset management industry?
What is artificial intelligence (AI)?
While there is no single, universally accepted definition, artificial intelligence (AI), or machine learning (ML) as a subset of AI, generally refers to the ability of machines to exhibit human-like intelligence and a degree of autonomous learning. An example would be machines solving a problem without the use of hard-coded software containing detailed programming instructions.
An artificial neural network is one of many computational algorithms used for the purpose of machine learning. Built with the intention of simulating biological neural systems, artificial neural networks come closest to the concept of an artificially engineered intelligence among all AI algorithms. In biological brains, electrochemical impulses transmit through a network of neurons that consist of a cell body, dendrites and axons. Dendrites are receptive extensions of a nerve cell that transport signals into the cell body which accumulates signals until a certain threshold is reached. Then, axons carry the electrochemical impulse away from the cell body to other neurons, muscles and glands.
Artificial neural networks emulate this behavior. While they do not match biological neural systems in complexity, they also consist of a web of artificial neurons, so called nodes, which are bundled in different layers that are interconnected. The nodes contain mathematical activation functions, configured to convert an input signal into an output signal. The learning done by these nodes can be understood as parametrization processes that take place in each activation function in every node. The machine tunes and tweaks a large amount of parameters until the input features received are converted into the predicted output. Once learning is complete, the machine is able to apply its knowledge to unknown data. The artificial brain literally learned how to perform a task.
As a result, artificial intelligence, enabled by adaptive predictive power and autonomous learning, dramatically advances our ability to recognize patterns in unstructured data, anticipate future events in financial markets and therefore make the right investment decisions.
Why AI has launched the next quant revolution?
Traditional quantitative models employ a large set of statistical tools aiming to mathematically capture the relationships between a set of financial variables, given very restrictive and often unrealistic assumptions. In particular, (i) one has perfect knowledge about the model’s specification (e.g., Gaussian distribution) and (ii) one knows all the variables involved in a phenomenon (including all interaction effects). In this way, although they might be a good first approximation of the true relationships, they miss out on what is truly valuable for asset and investment managers: the capacity to quickly adapt to changing conditions.
However, what appears to be the common pain point of traditional stochastic models is that they struggle to understand the structure of time-varying and complex relationships. On the one hand, under-complex models risk to provide only a few insights, as they will leave on the table most of the valuable information. On the other hand, over-complex models would closely mirror past observations with a high risk of overfitting, that is, to fail in generalizing the relationship and have a high error rate on new data sets.
Moreoever, traditional models have difficulty in exploring all the possible combinations of variables and therefore risk being unstable as time goes by and markets change. Low p-values are meaningless when researchers wrongly assume a linear relationship between variables, or omit interaction effects, e.g., there is no theoretical reason to expect a linear relationship between financial returns and risk factors, hence the low p-values found in the factor literature can be misleading. Classical econometrical models applied to financial series therefore often result in poor performance with a high bias, error caused by erroneous assumptions, and a high variance, error caused by overfitting.
On the contrary, AI models – such as ML and Artificial Neural Networks (ANN) – minimize overall estimation errors. They capture the regularities in the data (low bias) while generalizing well to unseen data (low variance). This is a key difference between Econometrics and ML. Econometric models are willing to sacrifice estimation performance in exchange for zero bias while ML models find a balance between bias and variance in order to maximize estimation performance. These ML methods can achieve lower Mean-Squared-Errors (MSE) than econometric models through “Cross-validation”, “Complexity reduction” (Regularization, Feature selection, dimensionality reduction) as well as “Ensemble methods” (Bootstrap aggregation / Bagging, Boosting, Stacking).
ML methods perform this task to scale, leveraging their high computational power to process each possible combination simultaneously so that they have a better understanding of the true interactions between the different components of the model. This makes AI particularly suited to processing massive amounts of data and to uncover hidden and complex interconnections between variables by capturing non-linear relationships and recognizing structural market shifts.
In addition, thanks to their continuous learning, AI models adapt to new data, learning without pre-established schemes, so to ensure that the knowledge acquired is always up to date, expanded and improved with the new observations.
To conclude, compared with traditional stochastic methods, AI tools enhance the out-of-sample predictability, they rely less on potentially unrealistic assumptions and they learn complex specificiations, including nonlinear, hierarchical and noncontinuous interaction effects. This results in an improved signal-to-noise ratio and a much better capacity to decipher the complex market dynamics.
Which types of AI data exist and what is its predictive power horizon?
In order to reliably build and train an AI model, the input data must not only be informative but also relevant for the investment horizon chosen to execute a particular strategy. The effectiveness of each type of financial data differs in terms of predictive power and predictive horizon. Predictive power is related to how much essential and useful information is aggregated in the data – that is, cause-effect relationships and underlying connections among assets. Predictive horizon refers to the time it usually takes for that information to be priced into securities and turned into exploitable investment opportunities.
Financial news and market sentiment represent a widely available source of information for many investors. However, when training the AI model, they risk adding noise and information that is only relevant for a short period of time. Since the predictive power of this data only holds for a very short time horizon, it is pivotal to process this data faster than competition and to employ this type of data only for short-term trading strategies.
Despite the potential of the unstructured and non-standard world of alternative data sets such as satellite images and social media posts, existing financial literature is still relatively heterogeneous regarding how much signal this data actually conveys. In this sense, the predictive power of alternative data is uncertain and therefore risks adding an extra layer to the analysis that blurs the real causality among asset dynamics. However, if employed in the right context alternative datasets have some sort of predictive power in the short-to-medium time horizon and can complement fundamental securities analysis.
Financial statements and fundamentals have long been the cornerstone of long-term value investing. Unfortunately, they present structural impediments that inhibit AI models from being responsive to changing market conditions, potentially missing investment opportunities that eventually disappear. However, sophisticated AI algorithms based on Natural Language Processing have been able to detect additional predictive information in the company statements, what resulted in an outperformance against the relevant market indices over a long-term investment horizon.
Historical market price data is the type of financial data that balances best the predictive power-horizon tradeoff. Indeed, asset prices are a standardized, indisputable and widely-available information source that ensures AI models to function efficiently and correctly. Market data is constantly updated, what greatly facilitates and improves the prediction power of AI, enabling investors to better extract the signal from the noise. It is to be noted, though, that competition in investment strategies, which rely solely on pure market price data analysis, is fierce and only investors with cutting-edge AI-technologies prevail.
To conclude, each type of dataset has its highest predictive power over its respective time horizon. Investors with sophisticated AI models which combine different types of data sets in their investment process have the best chances to generate a sustainable outperformance against their peers.
Which AI-methods can be applied to analyze price data (financial time series)?
Machine Learning as a subset of AI can be broadly referred to as a process where a system interacts with its environment in such way that the structure of the system changes, and that this interaction process itself changes as a consequence to structural alterations.
There are four main machine learning methods which each having different application areas in financial time series prediction.
Supervised learning is used for prediction tasks where a dataset with inputs and labeled targets is available. This may, for instance, entail using technical market indicators to predict whether the next day’s stock price will go up (1) or down (0), what is a binary classification. Apart from classification, supervised learning algorithms may also perform regression tasks, i.e. predicting a continuous value instead of a class label. Taking the stock price example above, this would translate to predicting the actual stock price or return instead of labeling winners and losers.
Unsupervised learning algorithms are usually designed for tasks that precede supervised learning, for instance, clustering or dimensionality reduction. An unsupervised learning algorithm may, for example, cluster stocks according to the similarity of their input features. The resulting cluster can then be further used for supervised classification or forecasting tasks.
Reinforcement learning is based on an action-response model over a multi-step chain of events. Reinforcement learning algorithms learn certain action policies which maximize expected rewards. Thus, they are highly applicable to environments where actions and rewards are clearly defined, such as board games. The reinforcement learning process is commonly based on a value function which expresses reward for an action undertaken at the current state of the system. In stock market forecasting, finding a suitable value function represents a major challenge, which is why deep reinforcement learning using back-propagation (feedback loop) with differential metric optimization objectives, has been widely applied. These methods learn from their loss function, for instance, “mean squared error” (MSE), which calculates the difference between the actual and predicted values. The derivative of the error function is then fed back through the network. Using this information, the algorithm adjusts the weights of each connection in order to reduce the value error function by some small amount.
Deep learning algorithms, an enhanced form of supervised learning, have evolved from simple multi-layer perceptrons to capture time dynamics through recurrent neural networks (RNN). In particular, gated neuron designs, which allow for capturing long-term dependencies in time series (e.g. Long Short-Term Memory (LSTM)), have gained popularity in order to explain complex, time-dependent, financial relationships. A common LSTM unit is composed of a cell, an input gate, an output gate and a forget gate. The cell remembers values over arbitrary time intervals and the three gates regulate the flow of information into and out of the cell. LSTM networks are well-suited to making predictions based on time series data, since there can be lags of unknown duration between important events in time series (“relative insensitivity to gap length”). RNNs using LSTM units partially solve the “vanishing gradient problem” of classic RNNs.
Can AI-powered algorithms predict financial time series?
With increasing competition and pace in the financial markets, robust forecasting methods are becoming more and more valuable to investors. Traditional statistics and econometrics are based on techniques first developed a couple of centuries ago and their applications in finance often involve linear regression models. These linear regression models assume normally distributed stock market returns and mostly fail in predicting these future returns.
The complexities in the real world are better captured using AI-techniques because of their ability to handle contextual and non-linear relationships, which can often arise in finance. For example, AI-techniques may be more effective than linear regressions in the presence of multicollinearity where explanatory variables are correlated. While machine learning algorithms offer a proven way of modeling these non-linearities in time series, their advantages against common stochastic models in the domain of financial market prediction are still based on limited empirical results. The same holds true for determining advantages of certain machine learning architectures against others.
However, evaluating the performance of machine learning algorithms in financial market forecasting show first convincing results:
„There is significant evidence for systematic outperformance of machine learning algorithms vs. traditional stochastic methods in financial market forecasting. These algorithms are able to capture meaningful non-linear dynamics in financial time series, and that these dynamics’ existence is generalizable across different market geographies and asset class prices. Furthermore, there is evidence that, on average, recurrent neural networks outperform feed forward neural networks as well as support vector machines which implies the existence of exploitable temporal dependencies in financial time series across multiple asset classes and geographies. Naturally, these findings have to be put into an appropriate context given the nature of prevailing research. There is no standardized dataset for machine learning algorithms in financial applications, as opposed to other popular application fields such as image recognition. Without norms regarding input data, extrapolation based on the performance of an algorithm for one market or one specific asset is impossible. Moreover, given that many machine learning algorithms exhibit a significant black-box characteristic and are highly sensitive to small changes in parameters, they are prone to data manipulation. As a consequence, there is a strong need for standardized training and testing procedures which will bolster comparability.“
(see paper of Ryll & Seidens, 2019)
Can AI improve the optimal portfolio construction?
Since the 50s, Markowitz’s modern portfolio theory (MPT) has been the most widely used approach for asset allocation and has inspired many extensions, including the Black-Litterman approach.
In practice, these convex optimization solutions deliver poor performance, to the point of entirely offsetting the benefits of diversification. For example, in the context of financial applications, it is known that portfolios optimized in-sample tend to underperform the naive (equal-weighted) allocation out-of-sample. The explanation for this underperformance is that the optimization framework is mathematically correct, but its practical application suffers from numerical problems. In particular, financial covariance matrices exhibit high condition numbers because of noise and signal. The inverse of those covariance matrices magnifies estimation errors, which leads to unstable solutions: Changing a few rows in the observations matrix may produce entirely different allocations. Even if the allocations estimator is unbiased, the variance associated with these unstable solutions inexorably leads to large transaction costs that can erase much of the profitability of investment strategies.
Financial correlation matrices are therefore not robustly estimated. For instance, a relatively small correlation matrix of only 100 instruments would require a minimum of 5’050 independent and identically distributed observations, or more than 20 years of daily data. Not only that, but correlations would have to remain constant for two decades, and returns would have to be drawn from a Gaussian process. Clearly, these are unrealistic assumptions.
In summary, these optimal MPT portfolios and their correlation matrices cannot be estimated robustly for two reasons: noise-induced instability and signal-induced instability. Machine learning techniques address the pitfalls of convex optimization in general and MPT in particular. According to M. Lopez de Prado and M. Lewis in their paper „Detection of false investment strategies using unsupervised machine learning methods“ a „nested clustered optimization“ (NCO) procedure shall be applied: (1) Cluster the correlation matrix using an ML algorithm like NCO; (2) apply the optimization algorithm to each cluster separately; (3) apply the optimal weights to collapse the correlation matrix to one row (and column) per cluster; and (4) apply the optimization algorithm to the collapsed correlation matrix. The optimal weights are the dot product of the weights in (2) and the weights in (4). The reason this procedure delivers robust weights is that the source of the instability is contained within each cluster, thus rendering a correlation matrix that is well behaved. (see paper M. Lopez de Prado and M. Lewis, 2018)
As a result, by having a probabilistic – rather than static – forecasting approach based on thousands of simulations, portfolio managers can forgo traditional backward-looking models and have a thorough understanding of the potential future shifts in correlations. Consequently, they can discount different future evolutions of the market and build more stable and efficient portfolios, which dynamically adapt to ever-changing market scenarios and eventually are better positioned to sustain unexpected shocks.
Does AI enhance the tools for financial risk management?
Markets in crisis mode are an example of how assets correlate or diversify in times of stress. It is essential to see how markets, asset classes, and factors change their correlation and diversification properties in different market regimes. The most recent crisis now adds to the list of scenarios available to risk managers, but it is still unknown what future crises will look like. Every crisis looks different because history rarely repeats itself. That is why simple backtests are problematic, being a view in the rear mirror only.
Therefore, it is desirable not only to consider real manifestations of market scenarios from history, but to simulate new, realistic scenarios systematically. To model the real world, AI-engineers turn to synthetic data, building artificially generated data based on so-called market generators. Market simulation of correlation matrixes unveils a new and flexible way of modeling financial time series.
Investment strategies could be systematically tested based on these simulations like a new car is systematically tested in a wind tunnel before hitting the road. In such environments, key system parameters are configurable. An investment strategy is said to be robust if it is not fragile in some situations. We could make specific assumptions regarding future scenarios, but if any of these assumptions do not hold, it will adversely affect the strategy.
As shown in the paper “Matrix evolutions – 2021 by Papenbrock & Schwendner” a novel method called “multiobjective evolutionary algorithm” is employed to generate realistic correlation matrixes of financial markets. The approach augments the training data space for an explainable ML program to identify the most critical properties in matrixes that lead to the relative performance of competing approaches to portfolio constructions. They show that the “Hierarchical Risk Parity” ML approach is very robust and that their method can identify the driving variables behind it. It produces realistic outcomes regarding stylized facts of empirical correlation matrixes and requires no asset return input data. It is suitable for parallel implementation and can be accelerated by graphics processing units (GPUs) and quantum-inspired algorithms. In this way, millions of realistic samples can be run to simulate correlated markets.
To conclude, matrix simulation should be used in risk management, such as in generating risk scenarios or new ways of (stress) testing multi-asset portfolios. This gives rise to a shift in the asset management industry toward a more mature, industrialized, digitized, systematic, and scientific way of constructing robust investment portfolios using the support of artificial intelligence (AI). With sound risk management practices, future regulatory requirements could be met. Examples are packaged retail investment and insurance products performance scenarios and European Securities and Markets Authority stress testing.
Can AI generate better option pricing estimates than Black-Scholes?
In 2018, the Chicago Board Options Exchange reported that over $1 quadrillion worth of options were traded in the US and a total dataset size of over 12 million examples of calls and puts can be retrieved from the exchange’s history. The vast majority of market practitioners are still relying on the option pricing model of Black-Scholes, which was developed in 1973. This model was derived by assuming stock prices are continuous and follow geometric Brownian motion, i.e. log-normally distributed returns, the riskless interest-rate and the volatility of the underlying asset are constant over the option life (i.e. the instantaneous volatility is nonstochastic), and that the market is frictionless (i.e. no transaction costs, short squeeze, taxes, etc.).
However, in reality Black-Scholes mismatches empirical findings and fails to explain the volatility surface (the so-called “volatitiy smile or smirk”), i.e. the implied volatility for both put and call options (of the same underlying asset) increases as the strike price moves away from the current stock price (i.e. away from at-the-money pricing). In other words, the implied volatility is not constant over time and strike prices. Over the last 45 years mathematically more complex computing approaches such as the Merton’s mixed diffusion-jump model (relaxing the continuous time assumption) or Rubinstein’s displaced diffusion model (relaxing the assumption of constant volatility), as well as Monte Carlo methods, all of them failed to beat the effectiveness of the Black-Scholes option-pricing equilibrium model.
Although AI-methods like “Recurrent Neural Networks” (RNNs) have been extensively applied to the stock price prediction problem, little work exists for volatility estimation and almost no work exists for options pricing. Despite the fact that neural networks have proven to be useful in modeling non-stationary processes and non-linear dependencies, only very recent ground-breaking research, carried out by Ke & Yang in 2019, found a large body of evidence that well-calibrated neural networks are able to achieve performance much superior to Black-Scholes in estimating the market price of options at closing.
As demonstrated in their paper (see research section: “Options Pricing and Deep Learning, 2019”) Multi-Layer Perceptron (MLP) or Long Short-Term Memory (LSTM) are the best suited neural network architectures considering option pricing and volatility estimation as a supervised learning problem. These neural networks, which use the same financial data as the Black-Scholes model, require no distribution assumptions and learn the relationships between the financial input data and the option price from the historical data during a training process.
As historical options data, the models use the same set of features like Black-Scholes such as underlying stock price, risk-free interest rate, exercise price, closing price, time to expiration as well as a naïve estimation of volatility (e.g. last 20 trading days). Since option pricing is a regression task, the objective is to minimize the mean squared error of the predictions.
First, a multilayer perceptron (MLP) is used to approximate the option price. This class of networks consists of multiple layers of computational units, usually interconnected in a feed-forward way. Each neuron in one layer has directed connections to the neurons of the subsequent layer. This MLP contains four hidden layers: three layers at 400 neurons each and one output layer with one neuron. The 400-neurons layers use Leaky ReLU as a rectified linear activation function, which is appropriate, since option prices are non-negative and non-linear. This MLP uses a learning technique called “back-propagation” (feedback loop). Here, the output values are compared with the correct answer to compute the value of some predefined error-function. The error is then fed back through the network. Using this information, the algorithm adjusts the weights of each connection in order to reduce the value of error function by some small amount. After repeating this process for a sufficiently large number of training cycles, the network converges to some state where the error of the calculations is small. In this case, the network has learned a certain target function. To adjust weights properly, one applies a general method for non-linear optimization that is called “gradient descent” (initialized with Glorot). For this, the network calculates the derivative of the error function with respect to the network weights and changes the weights such that the error decreases. For this reason, back-propagation can only be applied on networks with differentiable activation functions.
Thanks to the large training set, the risk of overfitting with only four hidden layers is controled and the model generalizes its findings. Eventually, this MLP model significantly improves over the Black-Scholes model in all error metrics and in particular for out-of-the money and illiquid options.
Second, Long Short-Term Memory (LSTM), an artificial recurrent neural network (RNN) architecture, is used to approach the option pricing problem. A common LSTM unit is composed of a cell, an input gate, an output gate and a forget gate. The cell remembers values over arbitrary time intervals and the three gates regulate the flow of information into and out of the cell. LSTM networks are well-suited to making predictions based on time series data, since there can be lags of unknown duration between important events in time series (“relative insensitivity to gap length”). RNNs using LSTM units partially solve the “vanishing gradient problem” of classic RNNs.
Because RNNs capture state information, this architecture can learn to estimate volatility from recent observations to improve option pricing performance. An 8-unit LSTM takes in the closing price at each timestep over 20 timesteps, chosen to calculate historical volatility over 20 days. The output sequence is fed forward for three layers of 8-unit LSTMs. The model consists of three LSTM layers that fed one output neuron.
To conclude, both neural network approximation methods achieve performance much superior to Black-Scholes using the same set of features, but by evading financial assumptions to view options pricing as a function.
Eventually, models could also be trained on the reverse problem to find the volatility implied by a given option price. This would allow to plot the volatility surface and compare it with the volatility surfaces from GARCH family models.
What is Natural Language Processing (NLP)?
Natural Language Processing (NLP) is the study and application of techniques and tools that enable computers to process, analyze, interpret, and reason about human language. NLP is an interdisciplinary field and it combines techniques established in fields like linguistics and computer science. These techniques are used in concert with AI to create chatbots and digital assistants like Google Assistant and Amazon’s Alexa.
In order for computers to interpret human language, they must be converted into a form that a computer can manipulate. However, this isn’t as simple as converting text data into numbers. In order to derive meaning from human language, patterns have to be extracted from the hundreds or thousands of words that make up a text document. This is no easy task. There are few hard and fast rules that can be applied to the interpretation of human language. For instance, the exact same set of words can mean different things depending on the context. Human language is a complex and often ambiguous thing, and a statement can be uttered with sincerity or sarcasm.
Despite this, there are some general guidelines that can be used when interpreting words and characters, such as the character “s” being used to denote that an item is plural. These general guidelines have to be used in concert with each other to extract meaning from the text, to create features that a machine learning algorithm can interpret.
Natural Language Processing involves the application of various algorithms capable of taking unstructured data and converting it into structured data. If these algorithms are applied in the wrong manner, the computer will often fail to derive the correct meaning from the text. This can often be seen in the translation of text between languages, where the precise meaning of the sentence is often lost. While machine translation has improved substantially over the past few years, machine translation errors still occur frequently.
Because Natural Language Processing involves the analysis and manipulation of human languages, it has an incredibly wide range of applications. Possible applications for NLP include chatbots, digital assistants, sentiment analysis, document organization, talent recruitment, and healthcare.
Chatbots and digital assistants like Amazon’s Alexa and Google Assistant are examples of voice recognition and synthesis platforms that use NLP to interpret and respond to vocal commands. These digital assistants help people with a wide variety of tasks, letting them offload some of their cognitive tasks to another device and free up some of their brainpower for other, more important things. Instead of looking up the best route to the bank on a busy morning, we can just have our digital assistant do it.
Sentiment analysis is the use of NLP techniques to study people’s reactions and feelings to a phenomenon, as communicated by their use of language. Capturing the sentiment of a statement, like interpreting whether a review of a product is good or bad, can provide companies with substantial information regarding how their product is being received.
Automatically organizing text documents is another application of NLP. Companies like Google and Yahoo use NLP algorithms to classify email documents, putting them in the appropriate bins such as “social” or “promotions”. They also use these techniques to identify spam and prevent it from reaching your inbox.
Groups have also developed NLP techniques to identify potential job hires and finding them based on relevant skills. Hiring managers are also using NLP techniques to help them sort through lists of applicants.
NLP techniques are also being used to enhance healthcare. NLP can be used to improve the detection of diseases. Health records can be analyzed and symptoms extracted by NLP algorithms, which can then be used to suggest possible diagnoses. One example of this is Amazon’s Comprehend Medical platform, which analyzes health records and extracts diseases and treatments. Healthcare applications of NLP also extend to mental health. There are apps such as WoeBot, which talks users through a variety of anxiety management techniques based in Cognitive Behavioral Therapy.
Which Deep Learning Models suit best for NLP?
Regular multilayer perceptrons are unable to handle the interpretation of sequential data, where the order of the information is important. In order to deal with the importance of order in sequential data, a type of neural network is used that preserves information from previous timesteps in the training.
Recurrent Neural Networks are types of neural networks that loop over data from previous timesteps, taking them into account when calculating the weights of the current timestep. Essentially, RNN’s have three parameters that are used during the forward training pass: a matrix based on the Previous Hidden State, a matrix based on the Current Input, and a matrix that is between the hidden state and the output. Because RNNs can take information from previous timesteps into account, they can extract relevant patterns from text data by taking earlier words in the sentence into account when interpreting the meaning of a word.
Another type of deep learning architecture used to process text data is a Long Short-Term Memory (LSTM) network. LSTM networks are similar to RNNs in structure, but owing to some differences in their architecture they tend to perform better than RNNs. They avoid a specific problem that often occurs when using RNNs called the exploding gradient problem.
These deep neural networks can be either unidirectional or bi-directional. Bi-directional networks are capable of taking not just the words that come prior to the current word into account, but the words that come after it. While this leads to higher accuracy, it is more computationally expensive.
Why NLP matters in asset management?
Natural language processing (NLP) by intelligent algorithms can extract value from fundamental and unstructured data on a company that comes in the form of annual or quarterly reports, earning transcripts, news reports, social media posts, emails and the like. By making inferences from language, machines can discern information on a specific company which allows it to make projections on the company’s revenue and cash flow streams. As long as this information is not fully priced in yet, profits can be made.
NLP can analyze every earnings call transcript in real-time in order to spot outliers, identify insights and understand key drivers. It assesses the relative predictive sentiment of individual transcripts and automated research reports of official corporate communication issues by thousands of companies. It can also analyze central bank announcements and it predicts the general market movements and trends based on the central bankers’ language.
The latest development of NLP is the so-called „Deception Model“ which is trained to parse every word of every sentence for the underlying financial meaning, but also to identify linguistic patterns that it has determined as being consistent with deceptive language. The model leverages a variety of techniques such as sentiment analysis, document classification, part-of-speech tagging, entity recognition, and topic detection in order to identify linguistic trends called „Events“ under the key driver, Deception. These events are made up of four components: omission (failure to disclose key details), spin (exaggeration from management and overly scripted language), obfuscation (overly complicated storytelling) and blame (deflection of responsibility). These „Deception Events“ may indicate evasive language, attempting to spin a positive sentiment with the analyst community on a negative event. To avoid biases, the model accounts for both the unique style of a given management team and the collective language of its industry peers (e.g., a young, overly optimistic technology CEO vs. a long-tenured oil exploration CEO). The Deception model can therefore detect the true meaning of the misinformation. The misinformation is then identified, tagged and exploited.
That these market inefficiencies can be exploited by NLP techniques is nicely demonstrated in the paper „Lazy Prices“ in which Prof. Lauren Cohen and Prof. Christopher Malloy explore the implications of a subtle “default” choice that firms make in their regular reporting practices, namely that firms typically repeat what they most recently reported. Using the complete history of regular quarterly and annual filings by U.S. corporations from 1995-2014 (K-10 reports), it is shown that when firms make an active change in their reporting practices, this conveys an important signal about the firm. Changes to the language and construction of financial reports have strong implications for firms’ future returns: a portfolio that shorts “changers” and buys “non-changers” earns up to 188 basis points per month (over 22% per year) in abnormal returns in the future. These reporting changes are concentrated in the management discussion (MD&A) section. Changes in language referring to the executive (CEO and CFO) team, or regarding litigation, are especially informative for future returns.
What are Convolutional Neural Networks (CNN)?
In 2012, a convolutional neural network (CNN) crushed all previous results at the largest image recognition competition. In the meantime, increased computational power, big data and a couple of key discoveries which reduced overfitting have further propelled the usage of CNNs. Today CNNs are state of the art image recognition models and have inspired approaches which systematize how chartists trade off from technical patterns. CNNs can “look” at price charts and are designed to learn to predict future returns for each chart.
A neural network operates by taking in data and manipulating the data by adjusting “weights”, which are assumptions about how the input features are related to each other and the object’s class. As the network is trained the values of the weights are adjusted and they will hopefully converge on weights that accurately capture the relationships between features.
This is how a feed-forward neural network operates, and CNNs are comprised of two halves: a feed-forward neural network and a group of convolutional layers.
A convolution is a mathematical operation that applies a local filter over an image. This filter consists of a set of weights and is called kernel. The filter that is created is smaller than the entire input image, covering just a subsection of the image. The values in the filter are multiplied with the values in the image. The filter is then moved over to form a representation of a new part of the image, and the process is repeated until the entire image has been covered.
Another way to think about this is to imagine a brick wall, with the bricks representing the pixels in the input image. A “window” is being slid back and forth along the wall, which is the filter. The bricks that are viewable through the window are the pixels having their value multiplied by the values within the filter. For this reason, this method of creating weights with a filter is often referred to as the “sliding windows” technique.
The output from the filters being moved around the entire input image is again an image, which is often called a “feature map”.
CNNs don’t use just one filter to learn patterns from the input images. Multiple filters are used, as the different arrays created by the different filters leads to a more complex, rich representation of the input image.
Why CNN can be employed to visualise fundamental or unstructured data?
In 2012, SEC mandated all corporate filings for any company doing business in the US to be entered into the Electronic Data Gathering, Analysis, and Retrieval (EDGAR) system. In finance, Generally Accepted Accounting Principles (GAAP) is a common set of accounting principles, standards, and procedures that companies in the US must follow when they compile and submit their financial statements. Researchers and rating agencies assess the credit worthiness of corporations based on these financial statements, and investors rebalance their portfolios based on the credit worthiness. Thus, understanding the fundamental relationship between accounting variables, financial ratios and corporate credit rating can help investors take informed decisions on modifying their portfolio.
Corporate financial reports are hard to analyze. Most rating agencies use a combination of relatively simple models with a lot of expertise assessments, as it is inherently difficult to associate hundreds of features contained in financial statements with a single credit rating score. For instance, the Compustat dataset, used to research US public companies, contains 332 financial data points per company issued on a quarterly basis. Immediate questions come to the mind: among the 332 are there any features significantly more important than others? Are those “important” features common for all industry sectors, or does the best feature set vary by sectors?
Feature selection could be one of the unsupervised machine learning techniques used to answer these questions. The goal of feature selection is to reduce the dimensionality of the input variable space and to improve the efficiency of statistical or learning models. Therefore, it is used to eliminate redundant, irrelevant, and noisy parts of the input data. It is generally argued that this process can improve the performance of classification models efficiently. In financial literature, the feature selection problem has been addressed using several techniques. For example, Principal component analysis (PCA), Chi Squared testing, genetic algorithm, and the Gini index.
In the paper “Is Image Encoding Beneficial for Deep Learning in Finance” by Wang & Florescu (see paper in the research section) it is concluded that, for the credit rating problem, it is better to use a Convolutional Neural Network (CNN) architecture on all available variables rather than using a traditional Multi-Layer-Perceptron (MLP) on the best selected feature subset. The CNN architecture, originally developed to interpret and classify images, is therefore also being used to interpret fundamental financial data due to its feature learning capability.
A typical CNN architecture will take advantage of the spatial relationship between neighboring pixels corresponding to parts of an object in an image. In a set of financial statements, a company’s fundamental data is categorized in balance sheets, income statements, and statements of cash flow. The features in the same category have closer relationships than features between different categories. For example, features in the balance sheet show what a company owns (assets) and owes (liabilities) at a specific moment, while features in the income statement show total revenues and expenses for a period of time. Furthermore, a lot of accounting variables are closely related. For example, it is commonly known that assets equal to the sum of liabilities and equity; and assets could be broken down into current assets and non-current assets. This is very similar to pixels forming up a particular object in an image.
It is proposed to use financial ratios such as valuation (P/E), profitiability (ROA), financial health (Cash Flow/Total Debt) or others (R&D/Sales) instead of raw fundamental data, since financial ratios are already a combination of original accounting variables. The way the data is transformed form fundamental to financial ratio data is similar to the way auto-encoders are supposed to work in artificial neural networks, i.e., dimensionality reduction by training the network to ignore singal “noise”. Using financial ratio data as input, the imaging methods produce significantly better results.
Unfortunately, financial datasets, particularly fundamental datasets are 1-dimensional data. In order to apply techniques developed for 2-dimensional spatial objects one has to encode the fundamental data into a 2-dimensional vector (matrix) by collecting variables in time. A so-called Recurrence Plot method can be applied to encode this type of time series fundamental data into a 2-dimensional image. There are different types of ways in which financial data can be “imaged”, i.e. transforming a vector into a matrix (“encoding”). The image is then used as input into a CNN model used for classification.
A CNN model can therefore be trained to identify the best features (financial ratios as “input” variables), which have the highest predictive power for corporate credit rating by Standard and Poor’s (as “output” variable). These best features vary by sector and industry. Once these best features are identified, they can serve as an intertemporal lead-indicator for a future change in the credit rating.
CNNs together with NLP can also analyze alternative data such as GPS and satellite imagery (e.g. agricultural data, rig activity, car traffic, ship locations, mining data) to see where consumers are going or to count cars in parking slots, business data (e.g. credit card data, store visit data, bills of lading, web search trends) to see what people are researching and talking about, as well as employee satisfaction data (e.g. social media, blogs, product reviews, tweets, cellphone location data).
As the volume of real-time data available increases beyond the capacity of individuals to analyze and understand it, the ability to compile, cleanse and evaluate that data automatically will be a key driver of performance in asset management.
How can Audio Signal processing filter the core information of financial time series?
Traditionally, the academic quantitative finance community did not have much overlap with the signal and information-processing communities. However, the fields are now seeing more interaction, and this trend is accelerating due to the intrinsically sequential nature of risk. Thus, machine learning and signal processing can be combined to solve high-dimensional mathematical problems with large portfolios and data sets.
In particular, the connections between portfolio theory and sparse learning /compressed sensing as well as between robust convex optimization and non-Gaussian data-driven risk measures through graphical models can be exploited.
When musician play together, their instruments’ sounds superimpose and form a single complex sound mixture. In the same way, the price signal is the aggregate of a large number of players / investors who get vocal and express their own view. The traditional music information retrieval is based on the decomposition of the audio signal into different elementary components which describe the harmonic structure of the music. Similarly, one can fit the harmonic structure of the daily returns signal using this decomposition paradigm, i.e., by introducing a set of elementary waveforms well localized in time, which one can correlate with the signal in order to capture the harmonic information over a short period of time.
As demonstrated in the paper “Low Turbulence” by Bramham Gardens (see research section) this approach explores an interpretable low-dimensional representation framework of financial time series combining signal processing, deep neural networks along with Bayesian statistics. This framework exhibits particularly good clustering properties, which leads to the definition of two market regimes: The High and the Low Turbulence regimes. By modeling their dynamics, one can forecast the market regime over the next period of time and adjust the market risk of a balanced portfolio accordingly.
The harmonic structure of the signal can be transformed into a series of spectral vectors. In order for a prediction model to make sense of it, one needs to reduce the complexity of the data even further. Describing complex features in a few dimensions is a well-known challenge in the computer vision community. The overly complex structure of the face image results from the intensity of each pixel composing the image, as well as from the correlations between the pixels.
By using autoencoder neural networks, one is able to map the whole picture into a low-dimensional representation called the bottleneck vector. One can think of each dimension of the bottleneck vector as a parsimonious description of the main facial features. The model is trained to reconstruct the face image based on the few desciptions provided by this bottleneck vector. This technique reduces dimensionality without losing the core of the price-series information. The compression process simplifies the complex multi-dimensional horizons / stress level picture into a low-dimensional risk vector.
As a result, the core intuition is that the price and volume of an asset summarizes very complex pieces of information. The technique described above therefore strives to de-noise the aggregate price signal at a sub-component level in order to only keep the core of the information in a recognizable manner. This kind of “de-noising” problem has actually been pretty well handled over time in the audio space where people looked to extract a recognizable compressed signal from a complex and noisy audio environment. There is now a large body evidence that this methodology also works for the risk prediction of financial time series.
How can Knowledge Graphs bring context to AI?
Knowledge Graphs enable machines to incorporate human expertise for making meaningful decisions and bring context to AI applications that go beyond the conventional machine learning approaches. Thus, the new wave of AI is focused on hybrid intelligence that means learning (data) fused with reasoning (knowledge). In other words, knowledge graphs combine two systems of thinking: System 1, which is powered by statistical AI approaches such as Machine Learning with System 2, which is powered by symbolic AI approaches such as Knowledge Representation & Reasoning.
Knowledge Graphs allow for processing and representation of data and knowledge in a format which is very close to the way a human brain processes and stores information. This enables users to quickly access two closely connected objects and adapt the connection based on the context, for example, let’s read the following sentence: “A red truck with a siren and a ladder attached to its side rushed in the city”. What do you understand from this sentence? As a human you might already think that the vehicle was most likely a fire truck; or there was probably a fire somewhere in the city; and many more logical conclusions. However, as a machine it would be very hard to make all those connections and come up with the above reasoning due to the lack of background and common-sense knowledge.
The impact of Knowledge Graphs in financial services is just in its inception where the role of knowledge scientists to build bridges between business requirements, questions and data is becoming more and more important. In finance, the knowledge graph is designed to find relationships amongst entities, such as the management of companies, news events, user preferences or supply chains. For this reason, the current research focuses on how to use knowledge graphs to improve the accuracy of stock price forecasts.
As an illustrative example let’s have a look at a Knowledge Graph model which dynamically captures and identifies the companies that are or will be market leaders in the field of carbon emission reduction. The model has a unique access to databases such as 10 Million+ patents, 100 Million+ scientific articles, 50 Million+ government contracts, 300k+ clinical trials, 5 Million+ court documents, 10 Million+ corporate official filings and 70 Million+ news articles of which it extracts an overwhelming quantity of unstructured and structured data.
From continuous and systematic reading of these documents combined with Natural Language Processing (NLP) and Deep Learning Network Theory the model establishes an extensive “Decarbonisation knowledge graph”. The knowledge graph represents a collection of interlinked descriptions of concepts, i.e., the knowledge graph puts the data into context via linking and semantic metadata and this way provides a multidimensional framework where relationships are grouped together.
Looking at electric cars a concept (“Electric cars”) is inextricably linked to another concept (“Tesla”). Tesla affects the demand for the chip technology which are produced by Nvidia. As a result, there is an inferred connection between Electrics Cars and Nvidia and that relationship is strong and positive through news flows, corporate transcripts, and price action.
To select the leading companies in the main theme “decarbonization”, the knowledge graph identifies a sub-theme/technology (e.g., Bio Diesel) which is strongly linked to “decarbonization” as well as the companies which are strongly attached to the sub-theme/technology through analyzing patents, filings, clinical trials, etc. As a result, there is an inferred connection between the theme “decarbonization” and the company.
To conclude, despite being in its infance, the Knowledge Graph methodology is one of the latest AI techniques to analyze complex and inter-connected data. In the paper “Anticipating stock market returns: A Knowledge Graph approach” by Liu & Zeng (2019), numerous experiments were conducted to derive evidence of the effectiveness of knowledge graph embedding for classification tasks in stock prediction. It is found that the Knowledge Graph approach achieves better performance than traditional stochastic approaches, other simple NLP methods, or convolutional neural networks.
Will AI change the asset management industry?
AI is particularly adaptable to securities investing because the insights it garners can be acted on quickly and efficiently. By contrast, when AI generates new insights in other sectors, firms must overcome substantial constraints before putting those insights into action. For example, when Google develops a self-driving car powered by AI, it must gain approval from an array of stakeholders before that car can hit the road. These stakeholders include federal regulators, auto insurers, and local governments where these self-driving cars would operate. Portfolio managers do not need regulatory approval to translate AI insights into investment decisions.
In the context of investment management, AI augments the quantitative work already done by security analysts in three ways:
First, AI can identify potentially outperforming securities by finding new patterns in existing data sets. For example, AI can sift through the substance and style of all the responses of CEOs in quarterly earnings calls of the S&P 500 companies during the past 20 years. By analyzing the history of these calls relative to good or bad stock performance, AI may generate insights applicable to statements by current CEOs. These insights range from estimating the trustworthiness of forecasts from specific company leaders to correlations in performance of firms in the same sector or operating in similar geographies.
Some of these new techniques produce significant improvements over traditional ones. In estimating the likelihood of bond defaults, for example, analysts have usually applied sophisticated statistical models developed in the 60s and 80s. Researchers have found that ML techniques are approximately 10% more accurate than those prior models at predicting bond defaults.
Second, AI can make new forms of data analyzable. In the past, many formats for information such as images and sounds could only be understood by humans; such formats were inherently difficult to utilize as computer inputs for investment managers. Trained AI algorithms can now identify elements within images faster and better than humans can. For example, by examining millions of satellite photographs in almost real-time, AI algorithms can predict Chinese agricultural crop yields while still in the fields or the number of cars in the parking lots of U.S. malls on holiday weekends.
A flourishing market has emerged for new forms of these alternative datasets. Analysts may use GPS locations from mobile phones to understand foot traffic at specific retail stores or point of sale data to predict same store revenues versus previous periods. Computer programs can collect sales receipts sent to customers as a byproduct of various apps used by consumers as add-ons to their email system. When analysts interrogate these data sets at scale, they can detect useful trends in predicting company performance.
Third, AI can reduce the negative effects of human biases on investment decisions. In recent years, behavioral economists and cognitive psychologists have shed light on the extensive range of irrational decisions taken by most humans. Investors exhibit many of these biases, such as loss aversion (the preference for avoiding losses relative to generating equivalent gains) or confirmation bias (the tendency to interpret new evidence so as to affirm pre-existing beliefs).
AI can be employed to interrogate the historical trading record of portfolio managers and analyst teams to search for patterns manifesting these biases. Individuals can then double check investment decisions fitting into these unhelpful patterns. To be most effective, individuals should use AI to check for bias at every level of the investment process – including security selection, portfolio construction and trading executions.
Will humans become obsolete in the asset management industry?
Yet despite the substantial enhancements to investment decisions, AI has its own limitations, which potentially undercut its apparent promise.
AI algorithms may themselves exhibit significant biases derived from the data sources used in the training process, or from deficiencies of the algorithms. Although AI will reduce human biases in investing, firms will need to have data scientists select the right sources of alternative data, manipulate the data, and integrate it with existing knowledge within the firm to prevent new biases from creeping in. This is an ongoing process that requires competencies many traditional asset managers don’t currently have.
Many of the patterns AI identifies in large data sets are often only correlations that cast no light on their underlying drivers, which means that investment firms will still need to employ skilled professionals to decide if these correlations are signal or noise. According to an AI expert at a large U.S. investment manager, his team spends days evaluating whether any pattern detected by AI meets all of four tests: sensible, predictive, consistent, and additive.
Even when ML finds patterns that meet all four tests, these aren’t always easily convertible into profitable investment decisions, which will still require a professional’s judgment. For example, by sifting through data of social media, AI might have been able to predict — contrary to most polls — that Donald Trump would be elected president in 2016. However, making an investment decision based on that prediction would present a difficult question. Would Trump’s election lead the stock market to go up, down, or sideways?
The bottom line is that while AI can greatly improve the quality of data analysis, it cannot replace human judgment. Human ongoing supervision is therefore key to ensure that AI is trained properly, starting from data collection and throughout the model’s continuous learning. To utilize these new tools effectively, asset management firms will need computers and humans to play complementary roles. As a result, firms will have to make substantial investments going forward in both technology and people, although some of these costs will be offset by cutting back on the number of traditional analysts.
Which asset managers will benefit from the AI revolution?
Unfortunately, most asset managers have not gone far down the path to implementing AI. According to a 2019 survey by the CFA Institute, few investment professionals are currently using the computer programs typically associated with ML. Instead, most portfolio managers continued to rely on Excel spreadsheets and desktop data tools. Moreover, only 10% of portfolio managers responding to the CFA survey had used AI techniques during the prior 12 months.
In the long-run, mid-sized asset managers should be able to benefit most from the AI revolution, because they are likely to attract and retain high-quality data scientists who may see more opportunities for advancement there than in the very large and rigid firms running uberly disintegrated IT infrastructure. In addition, these firms will be able to afford access to alternative data through third-party vendors, high-quality algorithms from open source libraries, and sophisticated tools from the technology companies (e.g., Amazon and Google) that already offering cloud-based services to many industries
The opportunity costs of not having implemented cutting-edge AI-technology to manage portfolios or assess risks are increasing day-by-day. As a consequence, asset managers, private banks and insurance companies will rather in-source the AI-technology from external experts and fintechs than building up entirely new AI-teams within their organization. On the one hand, it will be difficult to attract top AI-engineers to these “old-fashioned” companies and on the other hand, time is passing fast.
Is AI a new asset class for investors?
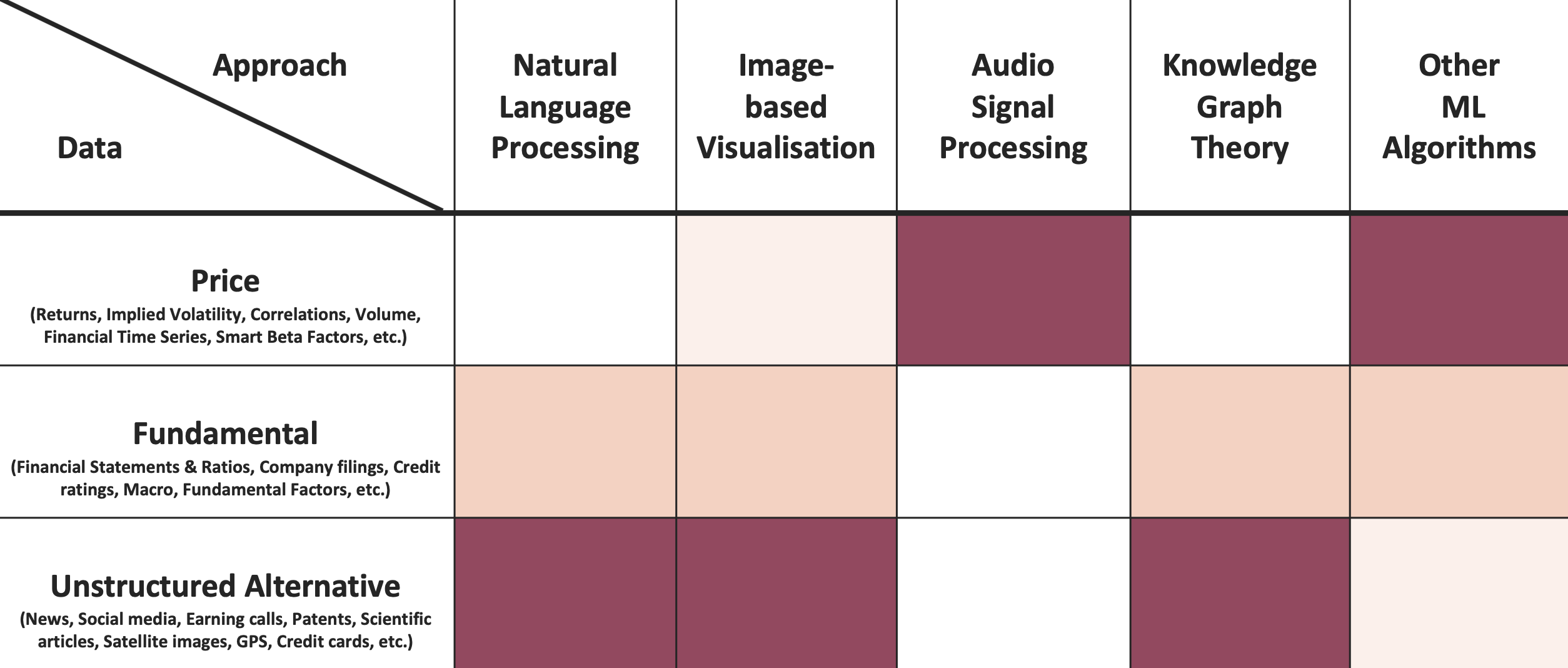
Taxonomy
AI-powered investment strategies can be classified into five Machine Learning approaches employing three different sets of data. A blank (white) field means that this technology cannot be applied to the respective data set. The more colourful a matrix field, the stronger the relationship between the ML-approach and the data sets.
It is important to note that all five ML-approaches might apply Deep Learning Artificial Neural Networks or Convolutional Neural Networks as a machine learning architecture to analyse the data. Some of these Deep Learning algorithms have evolved from simple multi-layer perceptrons to capture time dynamics through Recurrent Neural Networks. In particular, Gated Neuron Designs, which allow for capturing long-term dependencies in financial time series, text or speech analysis (e.g., Long Short-Term Memory (LSTM)), have gained popularity in order to explain complex, time-dependent relationships.
Most of the successful AI-powered investment strategies are specialised in one or a combination of the five ML-approaches applying one or more of the three data sets. Combining several investment strategies applying different ML-approaches in an investor’s portfolio delivers enhanced diversification effects.
Research Library
Paper
AI and big data in investment management
CFA Institute 2023
Survey
Evaluating the Performance of Machine Learning Algorithms in Financial Markets
Ryll & Seidens 2019
Paper
Detection of False Investment Strategies using Unsupervised Learning Methods
Lopez de Prado & Lewis 2018
Publication
AI Pioneers in Investment Management
CFA Institute 2019
Paper
Option Pricing with Deep Learning
Ke & Yang 2019
Paper
Anticipating Stock Market Returns: A Knowledge Graph Approach
Liu & Zeng & Yang 2019
Survey
The Future of Asset Management
Accenture 2021
Paper
Is Image Encoding Beneficial for Deep Learning in Finance
Wang & Wang & Florescu 2020
Paper
Lazy Prices
Cohen & Malloy & Nguyen 2015
Paper
Matrix Evolutions: Synthetic Correlations and Explainable Machine Learning for Constructing Robust Investment Portfolios
Papenbrock & Schwendner 2021
Survey
Transforming Paradigms – AI in Financial Services
University of Cambridge 2020
Paper
Predictable markets? A news-driven model of the stock market
Gusev & Govorkov & Sharov & Zhilyaev 2014
Publication
Knowledge Graphs for Financial Services
Deloitte 2021
Paper
Riskcasting Methodology
Bramham Gardens 2021
Paper
Deep Hedging of Derivatives Using Reinforcement Learning
Cao & Chen & Hull & Poulos 2020
Publication
Machine Learning in Finance
Deutsche Bank Markets Research 2016
Survey
Artificial Intelligence in Investing: Great aspirations – reverie or reality?
Vescore 2019
Publication
Artificial Intelligence: The next frontier for investment management firms
Deloitte 2019
Survey
Artificial Intelligence and Machine Learning in asset management
Black Rock 2019
Paper
Forecasting stock market returns over multiple time horizons
Kroujiline & Gusev & Ushanov & Sharov 2016
Book
Financial Signal Processing and Machine Learning
Akansu & Kulkarni & Malioutov 2016