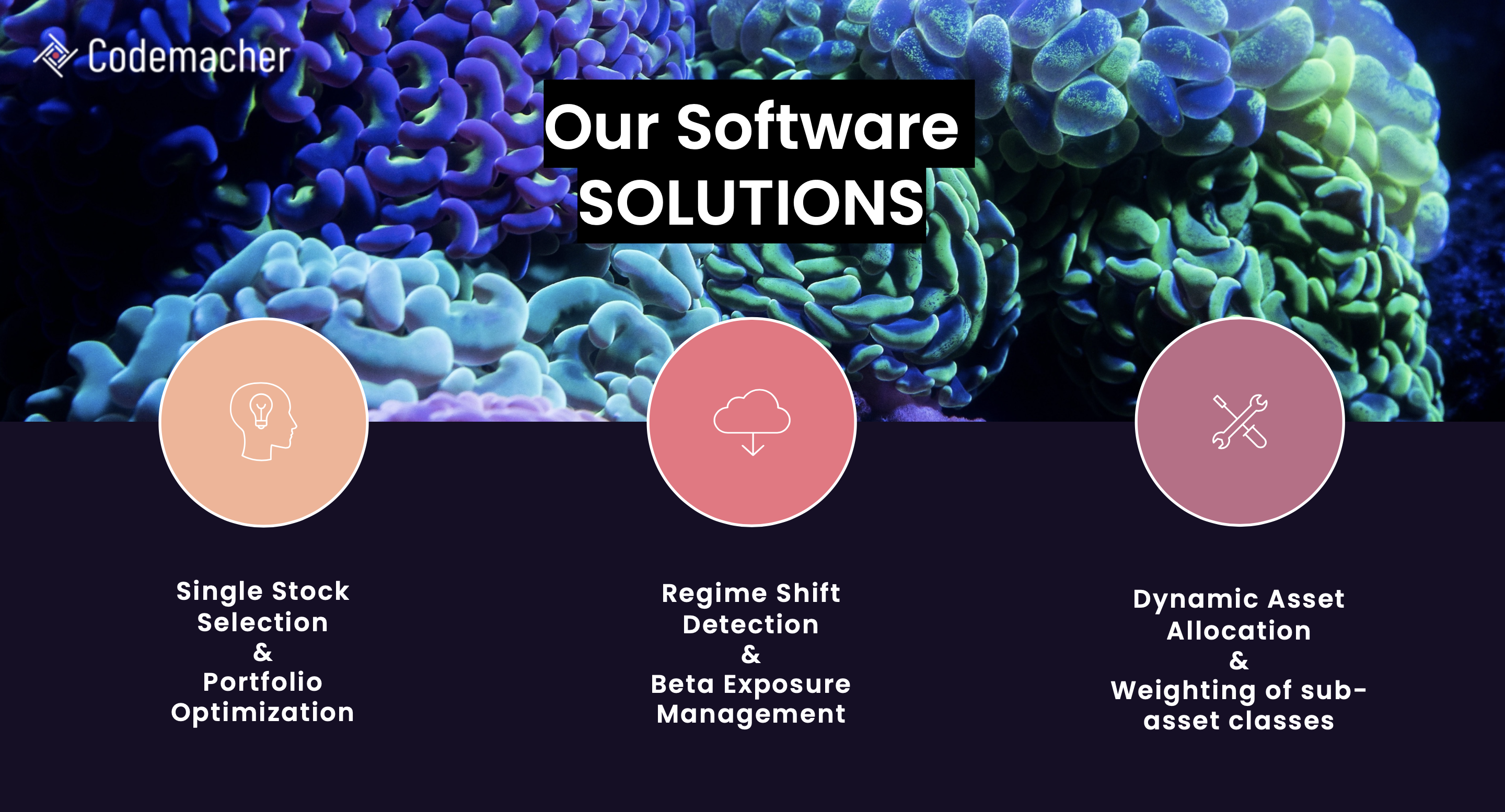
Our Solutions
We focus on software solutions and investment strategies whose intellectual property (IP) and algorithms are at the forefront of cutting-edge machine learning technologies.
These software solutions and investment strategies apply machine and deep learning neural network techniques using natural language processing (“NLP”), knowledge graphs, computer vision, and voice recognition to efficiently process market, text, image, and audio signal data.
These software solutions can be applied to assess the attractiveness of single stocks at a certain time, to detect early signs of a market regime shift and stock market correction, or to steer the tactical asset allocation dynamically.
The solutions impress investors by the explainable transparency of which features and factors drive the recommendations of the AI algorithms and they are fairly easy to integrate in the investment process of traditional investors through user-friendly web-based interfaces.
All solutions adhere to the UN-supported Principles for Responsible Investment (UNPRI), joining the leading international network of institutional investors committed to including ESG criteria in their investment process.
Strategies
We provide a business platform for exciting AI-powered software solutions and investment strategies with the finger on the pulse of groundbreaking next generation technologies. These strategies are implemented in either a traditional long-only or in an alternative investment approach. They cover the entire set of investable asset classes from equities over consumer loans fixed income to dynamic asset allocation solutions.
These innovative computer algorithms analyse unique sets of proprietary big data and generate consistent outperformance of their respective benchmarks while mitigating the risk of large drawdowns. Moreover, they constantly innovate, enlarge their datasets, enhance their algorithms and thereby come up with new inventions.
Interested investors are invited to fill in the form below in order to learn more about these software solutions and investment strategies. After filling in the form we will match the investor with the respective solution and upon request assist in the due diligence process.
Strategies
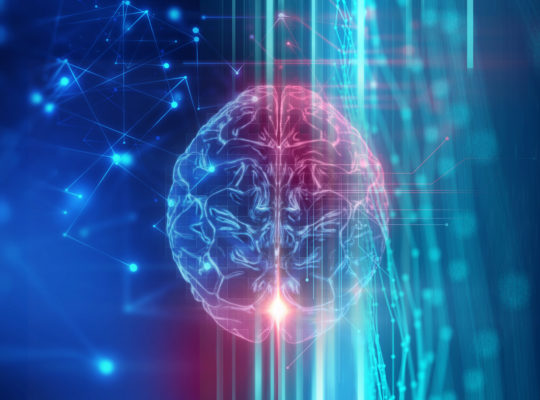
Strategy A
Fixed Income – US Consumer Loans
Strategy A has developed a proprietary loan selection methodology utilizing state-of-the-art machine learning algorithms to assess credit risk and to allocate capital to short duration, high yield credit in marketplace lending. The unsecured loans are self-amortizing in equal installments over 36 – 60 months and deliver an average return of 8-10% p.a. after fees. Strategy A possesses an exquisite network to source loans. It therefore surpasses the traditional „originator – seller“ market place lending platform model and employs an elevated loan underwriting model with direct access to the aggregator including proprietary selection and pricing filters.
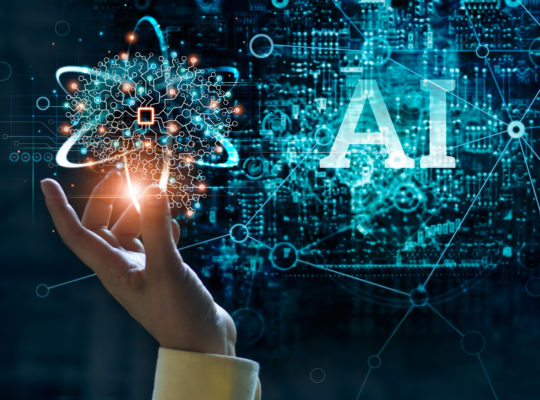
Strategy B
NASDAQ Global Equities Decarbonisation - long only
Strategy B’s AI model systematically selects the approximately 100 companies that are the most engaged in the transition to decarbonization within the NASDAQ Global universe (8’000 stocks). The technological innovations of these companies help to reduce and compensate for the emissions of carbon dioxide (CO2) and to achieve the long-term goal (2050) to create a CO2-free global economy. The transition is reinforced by policy and regulation in the European Union, in China and the United States. Multi-trillion budgets have been spoken in order to achieve the CO2-free world based on renewable energy technologies.
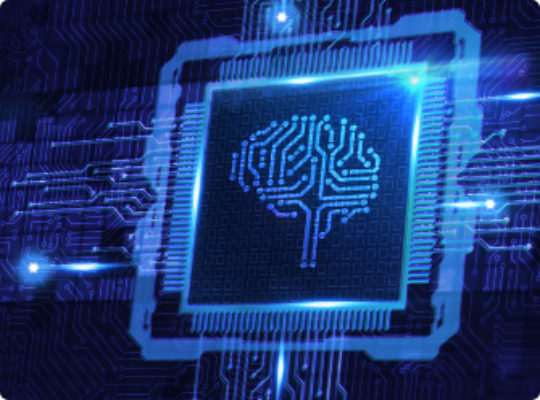
Strategy C
Multi Asset – Active Risk Management
Strategy C uses AI to track signals of investor views on market risk to determine three different market states: bullish, neutral, or bearish. It dynamically allocates between the S&P 500 equity index and the S&P 10-Year Treasury Note Futures Index based on a daily generated risk signal that reflects the market’s prevailing level of risk appetite. Traditional risk figures in finance tend to have backward-looking information on volatility, strategy C, however, looks at the imbedded risk information of over 20 million options contracts from S&P 500 options market in order to assess the current or near future risk sentiment.
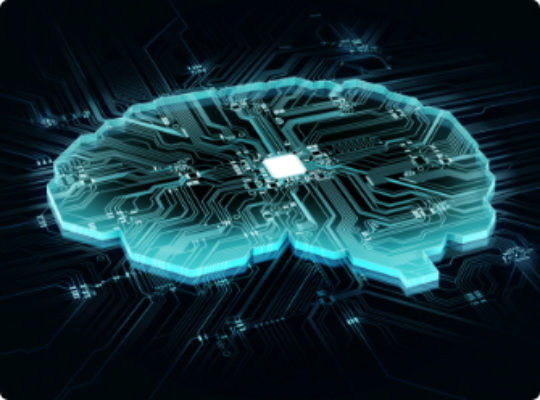
Strategy D
China Quant Equities - long only
Strategy D pursues an AI-powered quant equity long-only strategy in the Chinese A-shares stock market benchmarked to the CSI 300. The Chinese stock market is one of the most lucrative markets to generate alpha due to the high number of sentiment driven retail investors (up to 90% of trading volume) and due to the large number of alternative data sources available for data-driven investment strategies. Strategy D is available to overseas investors through brokerage accounts with „Stock Connect“, a unique collaboration between the Hong Kong, Shanghai and Shenzhen Stock Exchanges, which allows international investors to trade securities in each other's markets through the trading and clearing facilities of their home exchange.
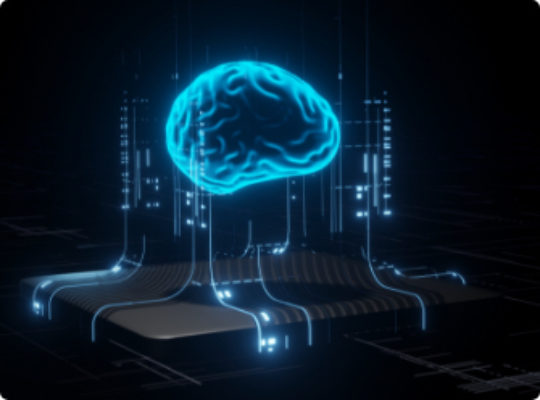
Strategy E
US Equities – Long / Short Statistical Arbitrage
Strategy E has developed machine learning algorithms which compile, cleanse, and analyze a large universe of traditional and alternative data sets with respect to the valuation of stocks. The strategy E assesses over 3'000 individual companies and sectoral trends and then enters into long or short positions to exploit mispricings.
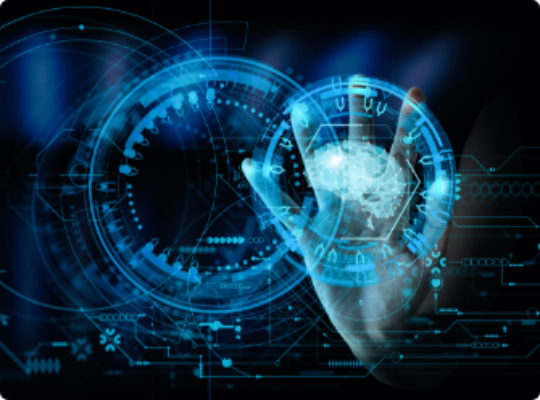
Strategy F
Global Macro – Signal Processing
Strategy F employs an AI algorithm based on the signal processing theory which automatically rebalances to the top 100 – 200 global opportunities across equities, bond, commodities and currency futures. The overall market exposure is risk-neutral, i.e. the positions in strategy F’s portfolio which are correlated to general risk-on market moves equal the positions which are correlated to general risk-off market moves on a volatility adjusted basis.
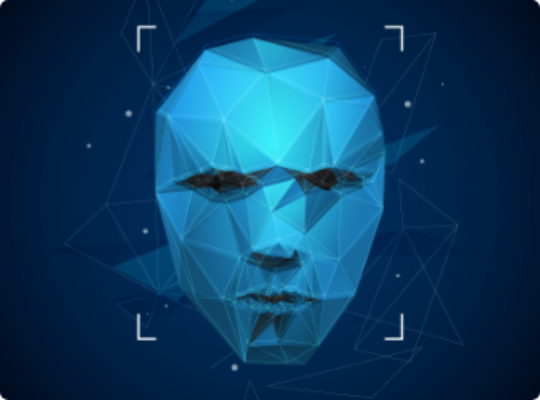
Strategy G
S&P 500 Equities ESG - long only
Strategy G’s AI algorithm systematically selects the 100 companies that rank the highest in terms of quality of Governance (ESG) within the S&P500 universe ex tobacco, defence, and oil companies. Companies that apply Good Governance typically outperform their peers and the reference index. Good Governance is forward looking and leads to superior Environmental and Societal values and is ultimately a reflection of the quality of top-level decision making. The Board as well as the Management of a company are key to deploy good governance practices; in this context the way the company communicates to the outside is a good way of capturing the quality of governance.
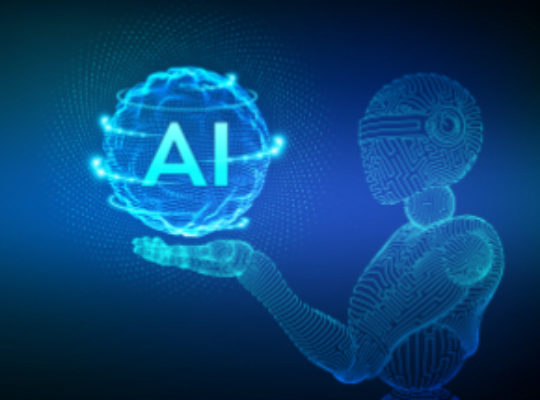
Strategy H
Global L/S Equities – Systematic News Based Trading
Strategy H employs news as the only data source for its AI-powered algorithms in order to understand investor sentiment dynamics and to scale up exposure to developed equity markets. It contains an array of independent trading signals that anticipate market movements in different equity markets over a 1-2 weeks time horizon and adjusts daily its long/short exposure in equity index futures. Mid-term (3-5 weeks) volatility risk forecasts are hedged via a long exposure in VIX futures. Long-term (several months) adverse market condition forecasts, which indicates an elevated bear market probability, are hedged via a “safe haven” basket of 10yr bonds and gold futures.
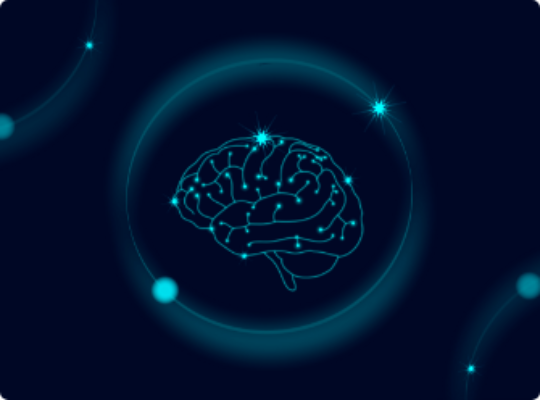
Strategy I
High Frequency Trading
Strategy i executes high-frequency trades through a suite of proprietary AI-powered technologies trading up to billions in daily volume across equity indices, interest rates, FX, commodities and metals.
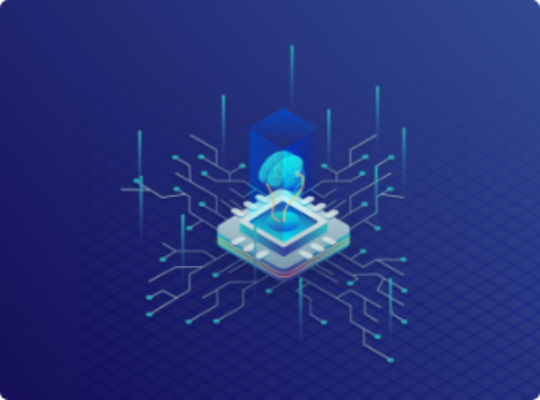
Strategy J
Managed Futures – CTA
Strategy J developed AI algorithms based on Bayesian Machine Learning (ML), Deep Learning (DL), Natural Language Processing (NLP) and multi-layer convolutional neural networks (CNN) for pattern recognition in order to trade futures and cash equities in various asset classes and geographies.
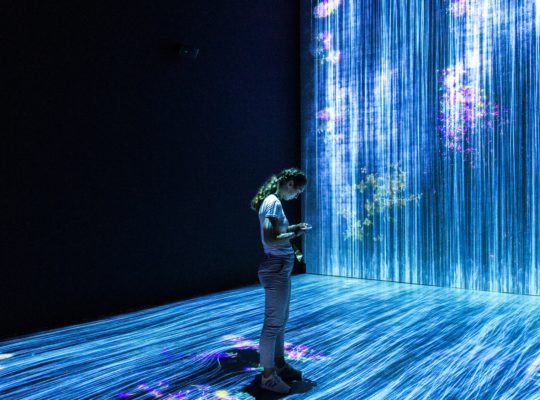
Strategy K
Fixed Income – China Corporate Debt
Valued at USD 13 trillion (as of December 2019), China’s growing debt market has a relatively short history and faces various disclosure issues. Strategy K developed an AI-powered credit evaluation engine that would allow it to value fixed-income instruments in real-time and to monitor developments of the broad market as well as individual issuers.
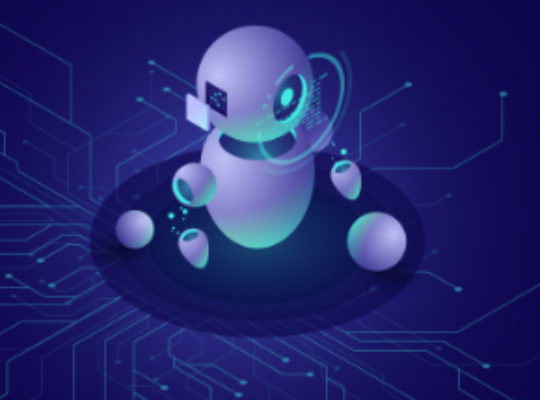
Strategy L
Private Equity Venture Capital - Direct investing platform
Strategy L is South East Asia’s leading platform for AI startup assessments, investments, and venture building. The assessment platform systematically detects “high traction AI tech startups”. These promising growth startups are selected through a transparent process which is a blend of multi-stage online evaluations conducted by seasoned global experts & proprietary ranking algorithms on the platform.
Strategy L has identified a global pool of 100+ early-stage AI enterprises, founded by industry practitioners that are serving mid-sized corporations solve specific business challenges and capture scaling opportunities using AI.
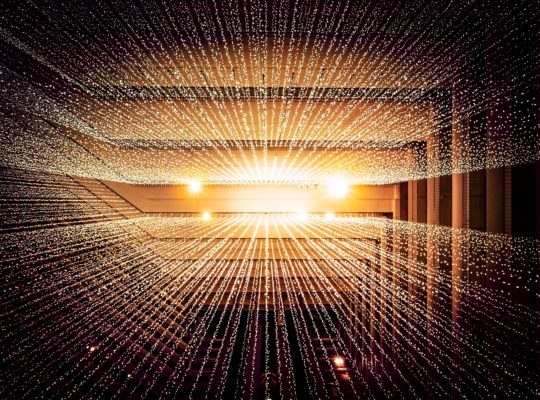
Strategy M
Global Macro – Diversified
Strategy M uses a non-trend following strategy combining 19 quantitative models grouped into three categories: (i) Behavioral (ii) Relative-Value and (iii) Macro
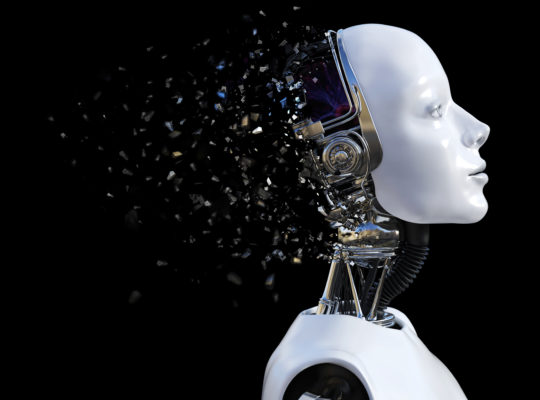
Strategy N
Multi-Asset - Balanced
Strategy N uses a highly sophisticated calculation system to compute large numbers of parameters and input combinations simultaneously. They use AI to analyse the results by visualizing thousands of multi-variate surfaces. By running millions of different configurations and scenarios Strategy N dynamically adjusts the optimal weights of a balanced multi-asset portfolio to the changing market environment. Strategy N thereby overcomes the shortfalls of modern portfolio theory which relies on unrealistic and static assumptions such as constant correlations and normally distributed returns.
Strategy A
Fixed Income – US Consumer Loans
How do AI applications enhance the strategy‘s performance?
The AI-powered algorithms gather the data from the national credit bureau and have the ability to evaluate more than 1500 credit attributes of each individual borrower and to identify mispriced US FICO scores of borrowers which rely on only 5 parameters on average.
US FICO scores and other traditional risk pricing underwriting tools therefore catalogue borrowers with highly discriminating features into similar risk and interest populations what leads to an inefficient assessment of the risk and return of each loan.
As it is public data, strategy A has access to more than 20 million loans with all the key attributes (parameters) and associated monthly loan performance data. The loan performance details record over billion data points, what is vastly sufficient for running highly sophisticated Deep Learning (DL) algorithms.
One of the big reasons ML and DL have been delayed in introduction in quantitative finance departments of large banks and mortgage credit companies is that the customary alternatives are accepted techniques such as linear and non-linear regression models for financial forecasting and logistic and survivability models for default risk. These rely on a 20-50 years-old body of mathematics where the essence can be explained by the firm to regulators in a moderate number of pages.
Traditionally, the same cannot be said of computational and data driven ML techniques. These models may embody thousands of decision points (as in random forests or gradient boosted trees), which renders any explanation a multi-page custom tour of the decision trees. AI techniques like DL take that to the extreme feeding data through multiple non-linear tensor transformations with millions of parameters derived iteratively from the training data. Simplifying the explanations of ML and DL classifiers has become important to simplify the complexity and making these more accurate techniques adoptable in practice.
Using DL and NLP techniques, strategy A has not only been able to simplify and explain the AI-models results, but also develop a robust predictive model around borrower characteristics in order to turn qualitative datapoints (e.g., job title), which all loan applications include, into a tokenized metric that can be used to better predict defaults. E.g., the algorithm found out that job titles such as „director“, „engineer“ or „attorney“ have a relatively lower default risk than job titles such as „driver“, „operator“ or „sales“. Moreover, it also identified that „lazy“ applicants, who write less or nothing in comment fields, have a higher tendency to default than others. It also detected the higher sensitivity of certain sectors to macro changes, e.g., a construction worker runs a much higher unemployment risk than a nurse during a prolongued recession and should therefore pay a higher risk premium than in standard risk pricing tools.
Since the loan data is updated on a monthly data, the AI-based risk management system dynamically assesses each individual loan and its interaction with key economic indicators in order to significantly reduce defaults. Loan defaults can exhibit signs of contagion raising the importance of geospatial analysis. The AI-based risk mitigation monitoring therefore shifts investments away from deteriorating credit quality in certain segments, increases the overall portfolio cash position during economic downturns with an increase in layoffs and late CC payments, pauses buying loans from a changing borrower population and applies pattern recoginition to identify abnormalities in borrower data.
Strategy B
NASDAQ Global Equities Decarbonisation - long only
How do AI applications enhance the strategy‘s performance?
Strategy B employs an AI-powered model which dynamically captures and identifies the companies that are or will be market leaders in the field of carbon emission reduction. The model has unique access to databases such as 10 Million+ patents, 100 Million+ scientific articles, 50 Million+ government contracts, 300k+ clinical trials, 5 Million+ court documents, 10 Million+ corporate official filings and 70 Million+ news articles of which it extracts an overwhelming quantity of unstructured and structured data.
From a continuous and systematic reading of these documents combined with Natural Language Processing (NLP) and Deep Learning Network Theory, the model establishes an extensive “Decarbonisation knowledge graph”. The knowledge graph represents a collection of interlinked descriptions of concepts, i.e. the knowledge graph puts the data into context via linking and semantic metadata and this way provides a multidimensional framework where relationships are grouped together.
Looking at electric cars a concept (“Electric cars”) is inextricably linked to another concept (“Tesla”). Tesla affects the demand for the chip technology which are produced by Nvidia. As a result, there is an inferred connection between Electrics Cars and Nvidia and that relationship is strong and positive through news flows, corporate transcripts and price action.
To select the leading companies in the main theme “decarbonization”, the knowledge graph identifies a sub-theme/technology (e.g. Bio Diesel) which is strongly linked to “decarbonization” as well as the companies which are strongly attached to the sub-theme/technology through analyzing patents, filings, clinical trials, etc. As a result, there is an inferred connection between the theme “decarbonization” and the company.
The knowledge graph has identified three major groups within the main theme “decarbonization”:
1) Efficiency Gains through smart grids, sustainable buildings, electric cars and energy storage
2) Energy Substitution through solar power, wind turbines, tidal/wave power, Bio Diesel and hydropower
3) Carbon Capture & Storage through pre-production carbon dioxide scrubbers or post-production carbon sequestration and ocean fertilization.
There are 25 sub-themes/technologies (e.g. solar power vs. wind turbines) within the three major groups which are independent to one another, but belong all to the overarching theme “decarbonization”.
For the portfolio construction Strategy B primarily focuses on the patent ownership as a main source of connection between the sub-theme/technology and the company. After a liquidity filtering the companies with the highest scores from other data sources (e.g. company filings) and with the highest number of positive news are eventually selected.
Strategy C
Multi Asset – Active Risk Management
How do AI applications enhance the strategy‘s performance?
Strategy C builds a volatility surface on a daily basis using the relevant option-related information from each day (maturity and strike price). The changes in the shape of this surface reveal insights about the market’s perspective on investment outcomes over differing future time periods.
Once this volatility surface is created, the first step is to compare the levels of return retrieved from the corresponding implied volatilities with the range of returns observed for the index itself over similar periods of time. From there, a risk-averse investor can extract a quantity akin to the extra remuneration required for holding some risk. The derived ratio is called the discount factor and expresses the price of the uncertainty of time, given the risk held. An extensive surface of these discount factors can be inferred, because different strike prices and maturities correspond to different risks.
The second step is to derive a surface of risk aversion levels, also known as relative risk aversion. This is accomplished using a closed-form deterministic methodology. This analysis focuses on measuring the changes in the discount factor surface into a risk aversion surface. A level of risk aversion can be associated, on a daily basis, with each strike and time horizon.
The consequences of these results are twofold. First, a daily measurement of investors’ dynamic risk attitudes can be retrieved from put and call options on the index. Second, the investor’s risk preferences can be clearly distinguished in relation to their time horizons and idiosyncratic market assumptions. Multiple time series of relative risk aversions per specific horizon and expected forward-market-price trigger (e.g. moneyness) are then derived.
In order to filter the time series properly, a technique called wavelet is applied.
In the next stage, a supervised machine-learning Bayesian classifier, which adapts to the data observed on a daily basis, is used to treat the output time series as high signal-to-noise input factors. These input factors subsequently determine whether current market conditions resemble those that preceded a significant rise or decline in the S&P 500. The combined risk signal is ultimately clustered broadly between bull, bear or neutral market stances and exhibit a high degree of stability from one day to the next.
Strategy D
China Quant Equities - long only
How do AI applications enhance the strategy‘s performance?
Strategy D employs three different types of machine learning methods depending on the nature of the data set and the desired output:
- Supervised learning methods such as Lasso, Ridge and Logistic Regression, Loess, K-Nearest Neighbor (KNN), Spline and Support Vector Machines (SVM) for well classified input features in order to predict non-linear short-term asset price direction, to find signals from noisy data or to use Gradient Boosting to predict short-term asset price direction based on macro data.
Strategy D also employs a Hidden Markov Model supervised classification in order to detect short-term regime shifts or to identify long-term market trends. - Unsupervised learning methods such as K-means, Birch, Gaussian Mixture Model (GMM) and Ward Spectral for the clustering of data.
Other unsupervised learning methods such as Principal Component Analysis (PCA), Independent Component Analysis (ICA) and Negative Matrix Factorization (NMF) for performing factor analysis in order to examine the entire set of asset returns and their relationships.
In contrast to supervised learning, unsupervised learning involves creating a model that is able to extract patterns from unlabeled data. In other words, the machine algorithm of strategy D analyzes the input features and determines for itself what the most important features and patterns are. It therefore tries to find the inherent similarities between different factors driving the stock market. - Deep Learning and Reinforcement Learning methods such as Convolutional Neural Networks (CNN), Multilayer Perceptron, Monte Carlo Tree Search (MCTS) or Cyclical Neural Networks to analyze time series or unstructured data (e.g. news or satellite images).
Strategy D thereby not only gets a fairly precise grasp of the current market sentiment, but also finds the most profitable trading signals by determining the optimal set and weights of factors (e.g. carry, value, momentum or market microstructure) which drive the stock market.
Strategy E
US Equities – Long / Short Statistical Arbitrage
How do AI applications enhance the strategy‘s performance?
First, strategy E has developed cloud-based text analytics algorithms using natural language processing (NLP) and machine learning (ML). It analyzes every earnings call transcript in real-time in order to spot outliers, identify insights and understand key drivers. Strategy E assesses the relative predictive sentiment of individual transcripts and automated research reports of official corporate communication issues by more than 3’000 companies. Strategy E is also specialized in analyzing central bank announcements and it predicts the general market movements and trends based on the central bankers language thereby steering the net market exposure of the overall portfolio.
Second, strategy E has recently developed a „Deception Model“ trained to parse every word of every sentence for the underlying financial meaning, but also to identify linguistic patterns that Strategy E has determined as being consistent with deceptive language. The NLP leverages a variety of techniques such as sentiment analysis, document classification, part-of-speech tagging, entity recognition, and topic detection in order to identify linguistic trends called „Events“ under the key driver, Deception. These events are made up of four components: omission (failure to disclose key details), spin (exaggeration from management and overly scripted language), obfuscation (overly complicated storytelling) and blame (deflection of responsibility). These „Deception Events“ may indicate evasive language, attempting to spin a positive sentiment with the analyst community on a negative event. To avoid biases, the model account for both the unique style of a given management team and the collective language of its industry peers (e.g., a young, overly optimistic technology CEO vs. a long-tenured oil exploration CEO). The Deception model can therefore detect the true meaning of the misinformation. The misinformation is then identified, tagged and exploited.
Third, strategy E analyzes alternative data such as GPS and satellite imagery (e.g. agricultural data, rig activity, car traffic, ship locations, mining data) to see where consumers are going or to count cars in parking slots, business data (e.g. credit card data, store visit data, bills of lading, web search trends) to see what people are researching and talking about, as well as employee satisfaction data (e.g. social media, blogs, product reviews, tweets, cellphone location data). The algorithms find patterns in this data through unsupervised deep learning techniques powered by computer vision like convolutional neural networks (CNN) and then generate trading signals for investment ideas. As the volume of real-time data available increases beyond the capacity of individuals to analyze and understand it, the ability to compile, cleanse and evaluate that data automatically is a key driver of performance.
Strategy F
Global Macro – Signal Processing
How do AI applications enhance the strategy‘s performance?
Strategy F combines machine learning and signal processing to solve high-dimensional mathematical problems with large portfolios and data sets. Traditionally, the academic quantitative finance community did not have much overlap with the signal and information-processing communities. However, the fields are now seeing more interaction, and this trend is accelerating due to the intrinsically sequential nature of risk.
In particular, strategy F exploits the connections between the portfolio theory and sparse learning /compressed sensing as well as between robust convex optimization and non-Gaussian data-driven risk measures through graphical models.
The core intuition of strategy F is that the price and volume of an asset summarizes very complex pieces of information. The technique therefore strives to de-noise the aggregate price signal at a sub-component level in order to only keep the core of the information in a recognizable manner. This kind of “de-noising” problem has actually been pretty well handled over time in the audio space where people looked to extract a recognizable compressed signal from a complex and noisy audio environment.
Strategy F relies on experts-in-imaging techniques to reduce dimensionality, without losing the core of the price-series information. The compression process simplifies the complex multi-dimensional horizons / stress level picture into a three-dimensional risk vector.
As a result, strategy F derives a probability density function, or a 3D map for each of the instruments, assigning probabilities to the future price of all the instruments over time.
Strategy G
S&P 500 Equities ESG - long only
How do AI applications enhance the strategy‘s performance?
Strategy G measures Good Governance with a proprietary AI methodology, which is based on the systematic analysis of the language (NLP) used by the companies in their 10-K reports.
In contrary to traditional governance measures which analyze simple parameters such as governance processes, executives’ bonuses, and accounting data, the model of strategy G takes into account multiple stakeholders (customers, owners, employees, suppliers, community environment, etc.) as well as emphasizes innovation, financial prudence and proactive risk management approaches in order to produce a “G” rating. As a result, strategy G produces a true and long-term value-based governance measurement.
The AI methodology relies on a proprietary dictionary of approximately 7’000 keywords in order to derive a Governance score which measures the quality of governance of a company. The model analyzes the language employed for the timeframe, the people and the stakeholders.
For the language of timeframe, companies with high governance scores use words such as “century, continue, constant, decade, era, future, long-term and perpetual” versus companies with low scores using words such as “currently, daily, immediate, month, overnight, promptly and quarterly”.
For the language of people, companies with high governance scores use words such as “career, colleagues, commitment, diversity, empower, grooming, promote, reward and spirit” versus companies with low scores using words such as “appraisal, assigned, dismiss, evaluated, hire, payroll, replaced, retention and wage”.
For the language of stakeholders, companies with high governance scores use words such as “carbon, child, climate, communities, culture, ecological, science and transparency” versus companies with low scores using words such as “appeal, attorney, claims, court, discharged, jurisdiction, litigation, petition, ruling and punitive”.
Strategy H
Global L/S Equities – Systematic News Based Trading
How do AI applications enhance the strategy‘s performance?
Strategy H’s return forecasting engine relies on ideas from statistical physics. It’s based on three steps:
First, financial news is scored using an in-house Natural Language Processing (NLP) methodology for textual analysis.
Second, the investor sentiment is calculated over multiple time horizons using a proprietary, physics-based model for sentiment dynamics.
Third, the modeled sentiments are applied to produce return forecast maps using a customized deep learning algorithm.
Strategy H has access to several hundred unique news sources from different data vendors. It collects tens of thousands news items for each equity market per day. The news data is downloaded every ten minutes (24h per day) and trading signals for each equity market are automatically generated.
The quality of the news input is constantly checked. If the signal dispersion (high forecast deviation) increases, the signal-to-noise ratio decreases or the sparseness (not fully connected layers of the artificial neural network) of the deep learning algorithm training sets gets too high, strategy H triggers an exit into a risk-neutral position.
Contrary to mainstream news-trading approaches, which focus on execution speed (minutes, hours) as a first linear price reaction to new information, strategy H’s model for mid-term (days, weeks) sentiment dynamics captures the non-linear relationship between new information and the long-term memory via interconnected news, sentiment and price.
Strategy’s H approach is underpinned by the latest academic research which demonstrates that the stock market is nearly efficient on intraday timescales, adjusting quickly to incoming news, but becomes inefficient on longer timescales, where news has a long-lasting non-linear impact on dynamics, attributable to a feedback mechanism acting over these horizons.
Strategy H’s approach offers therefore a scalable, regime-independent and uncorrelated alternative approach to investing in stock markets.
Strategy I
High Frequency Trading
How do AI applications enhance the strategy‘s performance?
Strategy i owes much of its edge to an emphasis on melding machine learning with low latency technology, i.e. there is a trade-off between speed and the trading quality. The strategy built their own feed handlers, which are systems that retrieve and organize market data from exchanges such as Nasdaq and Chicago Mercantile Exchange.
Strategy i has also developed its own order management system, which are instructions that determine how proprietary algorithms make trades. Strategy i’s system might gather over 300 million data points in the opening hour of the New York Stock Exchange on any given day. It can execute trades in just a few microseconds, and process data in mere nanoseconds.
Its machine learning algorithm identifies patterns in broker-dealer inventories, pricing data, and transaction costs. Based on these patterns strategy i executes trades to reduce latency, cost and market impact.
Using sequential machine learning in a high frequency trading context strategy i conducts huge numbers of statistical arbitrage trades seeking out a small edge on each trade and then make profits roughly 60% of the time. It searches for all sorts of sparse signals that could trigger a trade, like the speed or load of the market servers.
Securing servers in the Chicago Mercantile Exchange’s data center, writing a few million lines of code, and setting up the capability to support several petabytes of data, strategy i has built all the high frequency tools internally, including the servers, the colocation, the dark fiber channels and networks as well as all the execution software.
Strategy J
Managed Futures – CTA
How do AI applications enhance the strategy‘s performance?
Strategy J employs ML algorithms in order to map the economic cycle and to identify potential market regime shifts based on a wide range of incoming data on a day-to-day basis. These ML techniques are not only better to capture the true probability (non-normal) distributions of returns such as volatility, skewness (asymmetry) and kurtosis (fat tails), but also allow to incorporate far more data and a wider range of indicators than traditional stochastic measures.
Moreover, strategy J learnt from its specialized social media feeds algorithms (NLP), which issues real time alerts based on social media feeds and pre-runs short-term trends in various financial markets, when the king of Saudi Arabia died more than four hours before oil prices started to rise. Just recently strategy J has been alerted by its high-speed media feeds algorithm to an explosion at one of the largest gas hubs in the world before the story hit local and major news networks. The ensuing shutdown of the Baumgarten facility sent gas futures soaring. The strategy J capitalized on pre-entering the trade and by realizing gains after a short-term period.
In order to predict daily equity trading volumes segmented by markets strategy J employs a sequence-to-sequence model based on CNN and DL. The model is able to take in several time series of arbitrary length and output predicted series with the last element as the final prediction. The model covers 63 markets and has successfully anticipated several trade volume spikes (the model has an R2 of 0.86 for the US market).
The execution process of strategy J uses an adaptive intelligent routing algorithm to pick the optimum route to market for a given trade and takes into account the dynamically changing limit order book (LOB). Due to the nonstationarity and high degree of noise in transaction cost data using ML techniques, like reinforcement learning, in order to automatically allocate trades to one of the available execution channels (e.g. Algo A, Algo B, …, human) has proven to be the most efficient way.
Strategy J employs a specialist hardware – that is, a processor called a graphical processing unit (GPU) which completes the calculations required for DL research in 1/30th of the time taken by the CPU in a standard computer. It uses the programming language „Python“ for researching both, the trading and the execution algorithms.
Strategy K
Fixed Income – China Corporate Debt
How do AI applications enhance the strategy‘s performance?
Strategy K’s credit evaluation engine gathers publicly available information including company annual reports, financial statements, legal proceedings (lawsuits) and regulatory filings as well as data and news from websites, social media and third-party vendors regarding over 20’000 equity and debt issuers including the information of 1’700 municipal corporate debt issuers.
Strategy K’s engine checks tens of thousands of websites in a minute and collects millions of data points daily from news, bulletin boards, and blog sites as well as announcements.
The engine’s algorithm runs Natural Language Processing (NLP) that captures information from the dynamic unstructured text data collected for analysis and modeling. The model then performs named entity recognition analysis on issuers and products to determine whether the article really focuses on them or just mentions them. Text Convulutional Neural Networks (CNN) then perform sentiment analysis on these text samples with over 90% accuracy when identifying negative information. The negative information is fed into a logistic regression model in order to predict the likelihood of a default of this particular issue. If the likelihood passes a certain treshold, the system alerts the risk management of the portfolio management, which is then able to sell the credit position before the broad market takes notice of the inherent issues.
Furthermore, the credit evaluation engine employs a knowledge graph which puts this data into context via linking and semantic metadata. This way it leverages knowledge reasoning techniques and performs in-depth mining on related-party business relationships (e.g. supply chains), which is very helpful in preventing the transmission of credit risk to a related company of the monitored „troubled“ entity.
Strategy L
Private Equity Venture Capital - Direct investing platform
Which types of AI-based ventures are on the platform?
German-based “Agranimo” creates micro-climate intelligence data products to improve the efficiency of the food supply chain and city ecosystems. Their unique & precise algorithms based on in-field climate and soil data, can help reduce water and fertiliser consumption, forecast farm yields, and assess climate risks on cities and food supply chains.
Singapore-based “AtomBrush” is designing & building wall-climbing swarm robots to automate construction tasks like painting, surface preparation, cleaning etc. They solve the core problems in the industry like shortage of skilled workers, low productivity and worker safety.
Malaysia-based “Food Market Hub” uses disruptive technology to help Food & Beverage operators optimize and monitor food costs, wastage, production and inventory operations. They use AI to improve restaurant procurement and food cost. It helps to consolidate orders to achieve bulk purchase advantages directly from suppliers and allowing for smart automatic reordering.
US/Indian-based “Intentico” is a Cognitive Customer Experience (CX) platform which helps enterprises automate customer service issues within 60 seconds through its suite of products – AI speech bots, AI chatbots, middleware, bot ticketing & unified dashboard. Intentico’s mission is to create ‘Cognitive eXperience as a Service’ (CXaaS).
Spain- based “Kibus” is a first home AI appliance device that cooks healthy pet food automatically, using multi-dose capsules of human-grade dehydrated food. It allows pet parents to feed their pets on a healthy and natural diet in a convenient way, keeping the nutrients and flavours from the fresh ingredients. It has a great taste and it has been proven that feeding pets on a minimally processed diet expands their lifespan by more than 30%.
Bangladesh-based “Jatri” is a ‘first-in-class’ mobile-as-a-service platform aiming to be the first journey planning solution for intra-city bus commuters in Bangladesh. The vision to extend this technology across all intra-city public transport modalities with a diverse range of smart solutions like digital tracking, digital ticketing, fleet management, order management, and network planning that enables passengers, operators and regulators to build a more efficient and sustainable public bus transportation network.
Singapore-based MindFi helps APAC companies deliver evidence-based personalised mental wellness to their employees, with award-winning mobile apps, analytics-driven and expert-led training model. MindFi advocates a fresh and practical approach for busy people to learn mindfulness meditation. They are different from their competitors in their AI-personalized approach, mobile apps, content and trainer localization for Asian clients.
Italy-based “THEMIS” has developed a high technological content operating in the White & Green biotechnologies sector. They have created a brand-new technology for the drastic reduction of organic waste (food waste, sludges, wastewater) and water recycling with unparalleled performances and multiple advantages. Themis WRT innovative and patented technology can reduce the original waste volume by 90-70%, returning 10-30% in the form of a re-usable treated solid product and the remainder in distilled water.
Strategy M
Global Macro – Diversified
How do AI applications enhance the strategy‘s performance?
Strategy M’s seven behavioral models anticipate the trades of large market participants using classical strategies, e.g. trend-following or carry. The AI-powered models dynamically forecast the signals used by these participants to enter into positions a few days or weeks ahead of them, therefore benefitting from their future market impact.
The G10-FX Mean-Reversion model learns the short-term dynamics of FX pairs using a projection method related to Principal Component Analysis (PCA).
The G10-FX Oil Dynamics model dynamically learns the relationships between crude oil and FX pairs. The model then uses one of the strongest signals on oil to forecast the pairs with significant linkage to oil.
A newly released model uses a similar approach to forecast metals based on a crude oil signal.
A current research project aims at finding recurring patterns in data, time series and non-structured data, to take profit from ‘hidden’ inefficiencies.
Strategy N
Multi-Asset - Balanced
How do AI applications enhance the strategy‘s performance ?
Strategy N uses AI and Machine Learning techniques such as Random Forests (multi-dimensional decision trees) to select the features and to compute how much each variable is contributing to the decision as well as Deep Neural Networks to determine the optimal weights of these input variables by comparing multi-variate surfaces with each other (clustering). Those techniques allow Strategy N to extract more signal out of the input data and build flexible models that adapt over time to new market conditions.
Strategy N leverages AI to exploit market inefficiencies, i.e. systematic anomalies in the (relative) returns of securities and asset classes that are the result of the interplay between biased behaviour from investors and the microstructure of financial markets.
Strategy N thereby not only gets a fairly precise grasp of which factors (e.g. carry, value, momentum, alternative risk premia or a new factor) currently drive financial markets, but also finds the most efficient portfolio construction in order to balance return expectations with risk.
As a result, by having a probabilistic – rather than static – forecasting approach based on millions of simulations, Startegy N can forgo traditional backward-looking models and has a thorough understanding of the current as well as potential future shifts in correlations. Consequently, Strategy N can discount different future evolutions of the market and build more stable and efficient financial covariance matrices, which dynamically adapt to changing market scenarios and eventually are better positioned to sustain unexpected shocks. The multi-asset portfolios based on Strategy N’s AI technology are therefore more robustly estimated and outperform naïve portfolios in the mid-to long-term.